AVFF: Audio-Visual Feature Fusion for Video Deepfake Detection
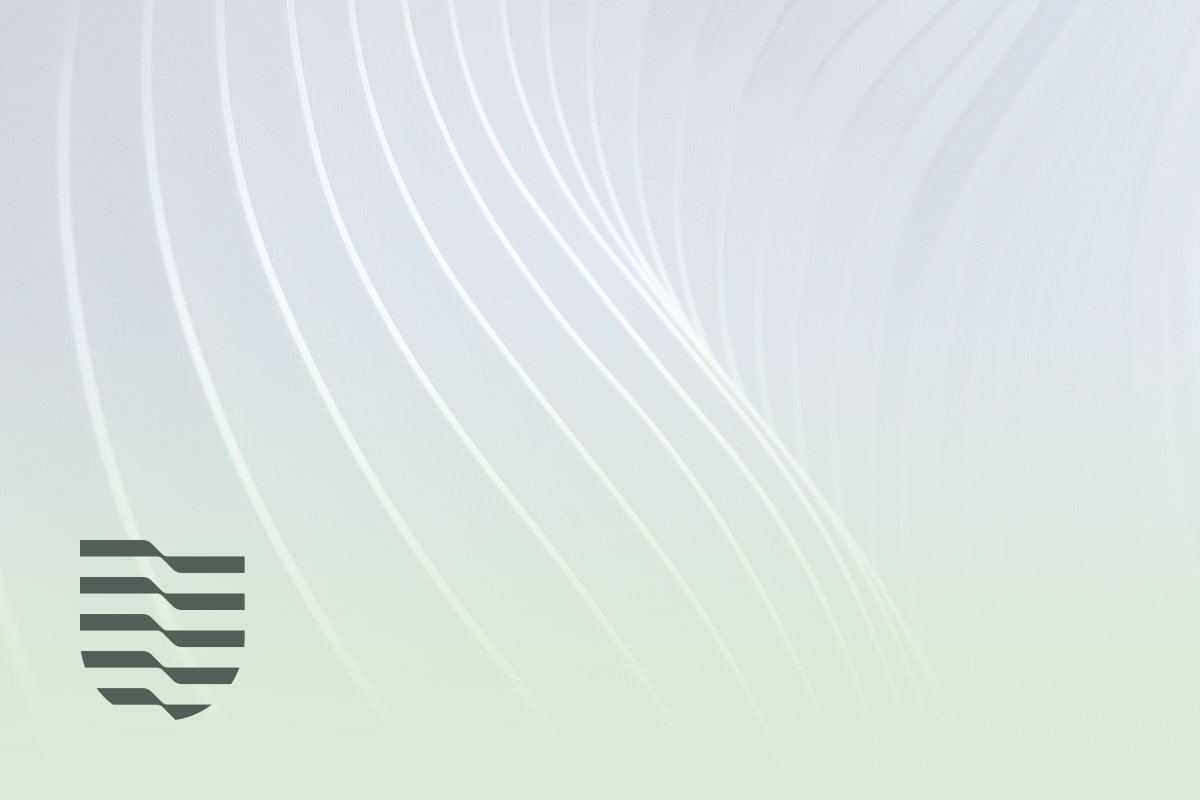
Summary
With the rapid growth in deepfake video content, we require improved and generalizable methods to detect them. Most existing detection methods either use uni-modal cues or rely on supervised training to capture the dissonance between the audio and visual modalities. While the former disregards the audio-visual correspondences entirely, the latter predominantly focuses on discerning audio-visual cues within the training corpus, thereby potentially overlooking correspondences that can help detect unseen deepfakes. We present Audio-Visual Feature Fusion (AVFF), a two-stage cross-modal learning method that explicitly captures the correspondence between the audio and visual modalities for improved deepfake detection. The first stage pursues representation learning via self-supervision on real videos to capture the intrinsic audio-visual correspondences. To extract rich cross-modal representations, we use contrastive learning and autoencoding objectives, and introduce a novel audio-visual complementary masking and feature fusion strategy. The learned representations are tuned in the second stage, where deepfake classification is pursued via supervised learning on both real and fake videos. Extensive experiments and analysis suggest that our novel representation learning paradigm is highly discriminative in nature. We report 98.6% accuracy and 99.1% AUC on the FakeAVCeleb dataset, outperforming the current audio-visual state-of-the-art by 14.9% and 9.9%, respectively.

Other Research
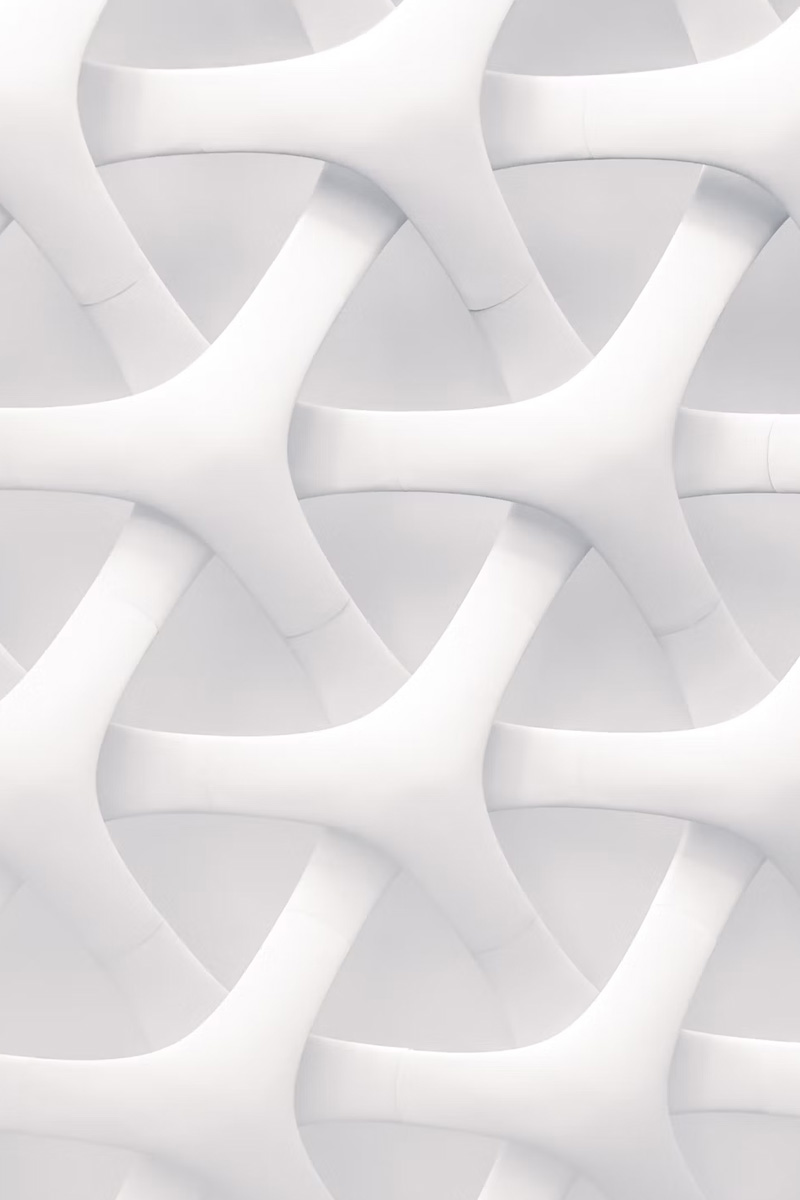
Learn from Real: Reality Defender’s Submission to ASVspoof5 Challenge
Published on
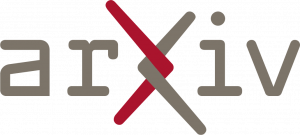

Patent: Identification of Neural-Network-Generated Fake Images
Published on
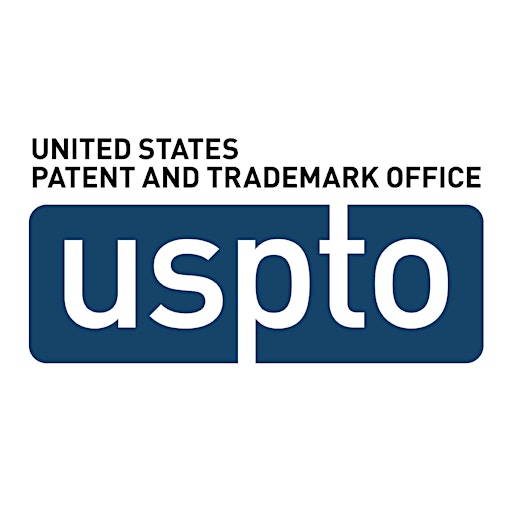
Subscribe to the Reality Defender Newsletter
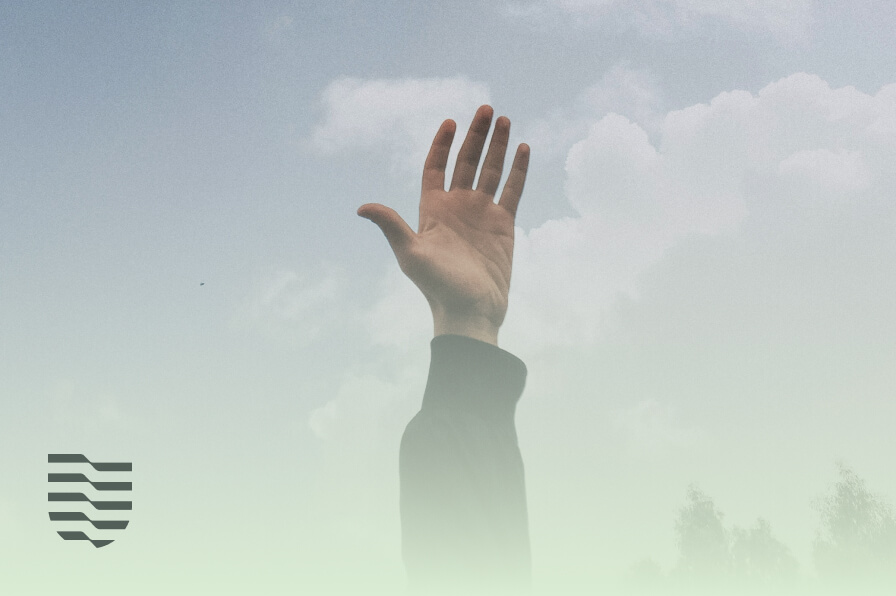