Preprint: Common-Sense Bias Discovery and Mitigation for Classification Tasks

Summary
Machine learning model bias can arise from dataset composition: sensitive features correlated to the learning target disturb the model decision rule and lead to performance differences along the features. Existing de-biasing work captures prominent and delicate image features which are traceable in model latent space, like colors of digits or background of animals. However, using the latent space is not sufficient to understand all dataset feature correlations. In this work, we propose a framework to extract feature clusters in a dataset based on image descriptions, allowing us to capture both subtle and coarse features of the images. The feature co-occurrence pattern is formulated and correlation is measured, utilizing a human-in-the-loop for examination. The analyzed features and correlations are human-interpretable, so we name the method Common-Sense Bias Discovery (CSBD). Having exposed sensitive correlations in a dataset, we demonstrate that downstream model bias can be mitigated by adjusting image sampling weights, without requiring a sensitive group label supervision. Experiments show that our method discovers novel biases on multiple classification tasks for two benchmark image datasets, and the intervention outperforms state-of-the-art unsupervised bias mitigation methods.
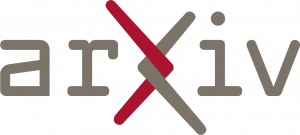
Other Research
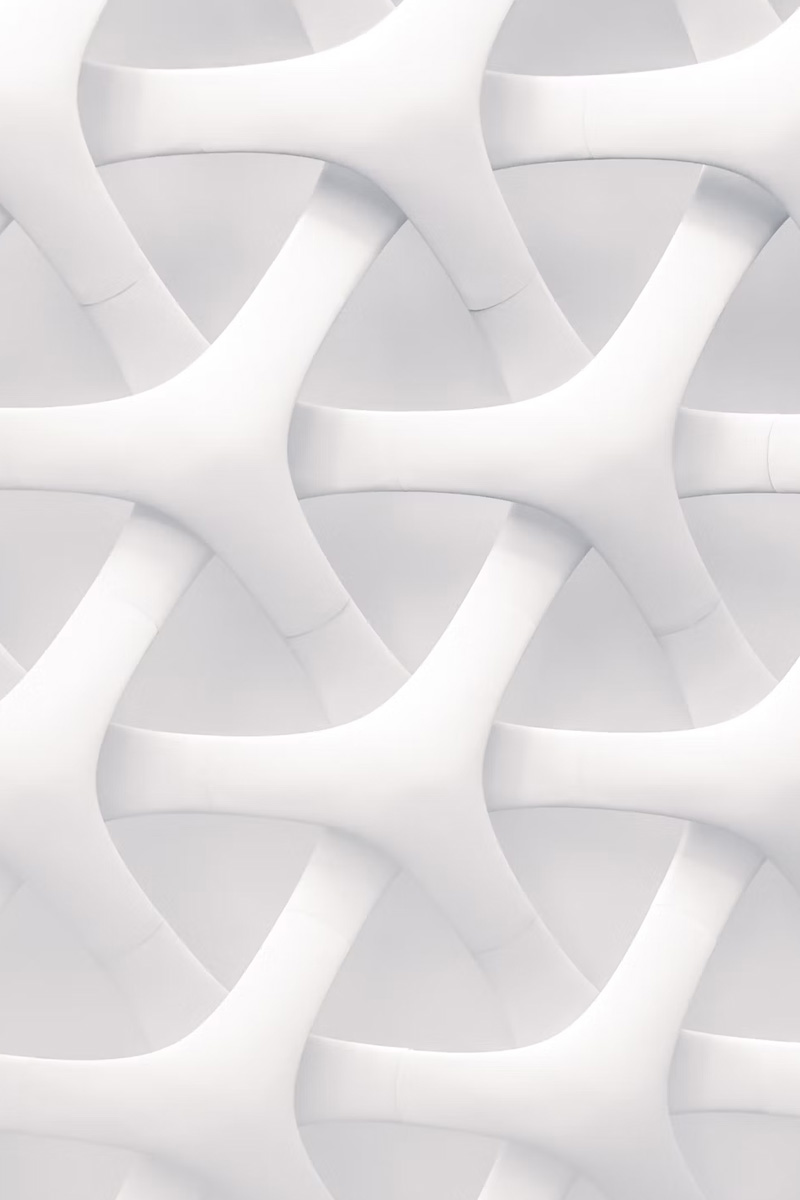
Learn from Real: Reality Defender’s Submission to ASVspoof5 Challenge
Published on
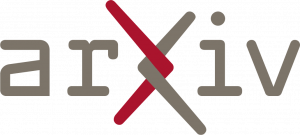

Patent: Identification of Neural-Network-Generated Fake Images
Published on
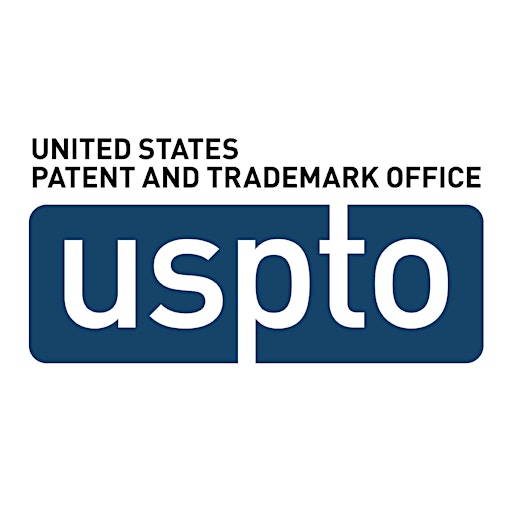
Subscribe to the Reality Defender Newsletter
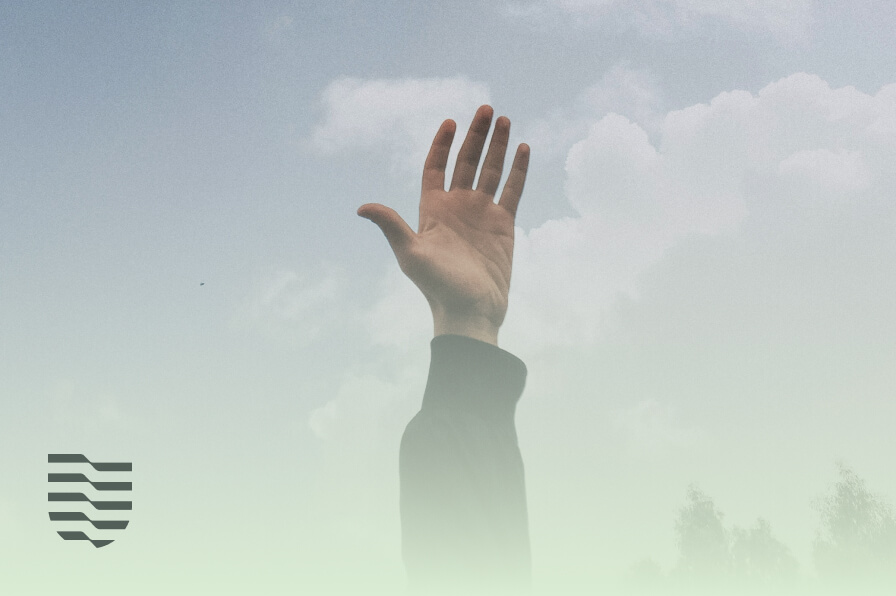