Generalized Spoofing Detection Inspired from Audio Generation Artifacts

Summary
State-of-the-art methods for audio generation suffer from fingerprint artifacts and repeated inconsistencies across temporal and spectral domains. Such artifacts could be well captured by the frequency domain analysis over the spectrogram. Thus, we propose a novel use of long-range spectro-temporal modulation feature -- 2D DCT over log-Mel spectrogram for the audio deepfake detection. We show that this feature works better than log-Mel spectrogram, CQCC, MFCC, as a suitable candidate to capture such artifacts. We employ spectrum augmentation and feature normalization to decrease overfitting and bridge the gap between training and test dataset along with this novel feature introduction. We developed a CNN-based baseline that achieved a 0.0849 t-DCF and outperformed the previously top single systems reported in the ASVspoof 2019 challenge. Finally, by combining our baseline with our proposed 2D DCT spectro-temporal feature, we decrease the t-DCF score down by 14% to 0.0737, making it a state-of-the-art system for spoofing detection. Furthermore, we evaluate our model using two external datasets, showing the proposed feature's generalization ability. We also provide analysis and ablation studies for our proposed feature and results.

Other Research
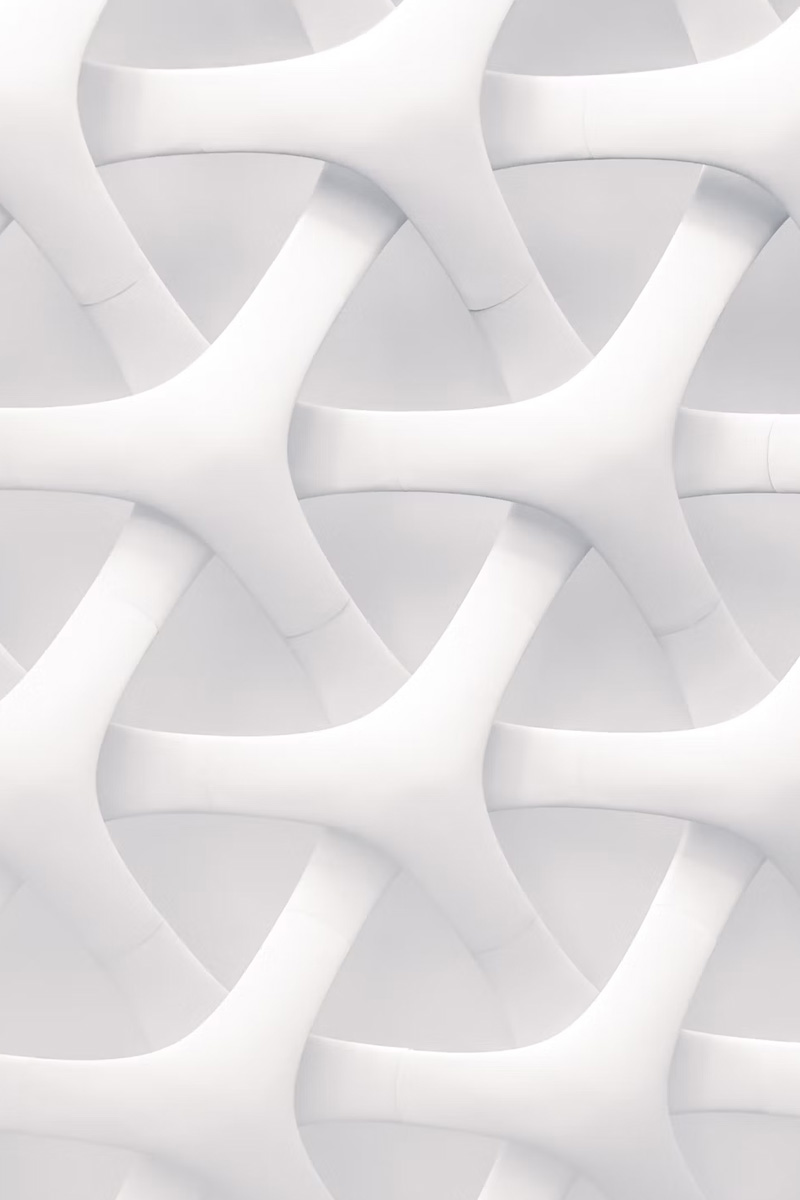
Learn from Real: Reality Defender’s Submission to ASVspoof5 Challenge
Published on
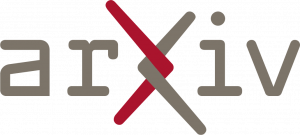

Patent: Identification of Neural-Network-Generated Fake Images
Published on
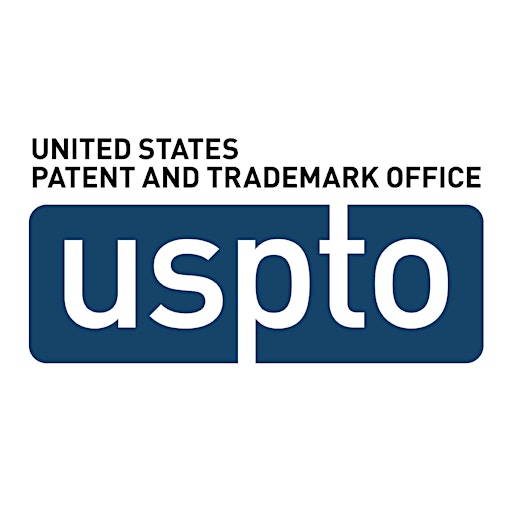
Subscribe to the Reality Defender Newsletter
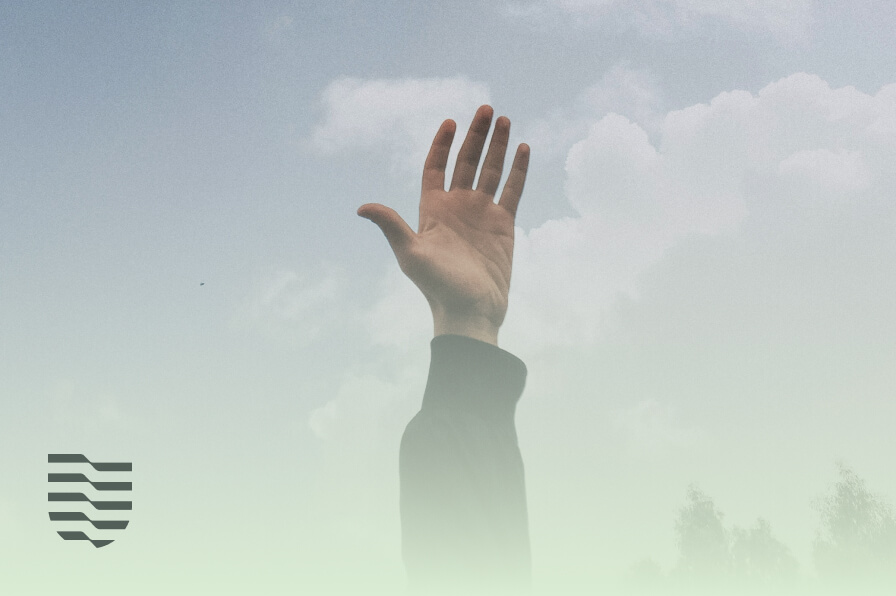